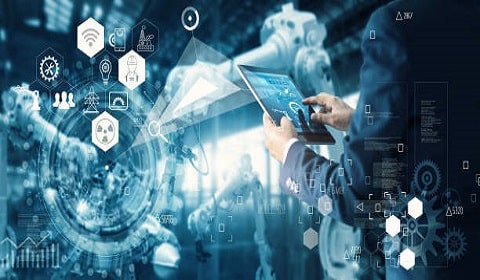
Top 10 Most Uses AI Tools for Data Analysts
AI tools for data analysts empower organizations by converting raw data into actionable insights, driving informed decision-making. Integral to data-driven enterprises, these tools offer accessibility to professionals of all backgrounds. Through visualization, analysis, and tracking capabilities, they facilitate the transformation of data into valuable business insights. This enables companies to achieve their objectives effectively, leveraging the power of data analytics to stay competitive in today’s dynamic market analysis.
AI Tools for Data Analysts
AI revolutionizes data analytics, offering potency, efficiency, and accessibility. It delves into vast data troves, unveiling crucial trends and patterns for enhanced business processes. By consolidating data streams, AI provides holistic insights, empowering users with comprehensive perspectives. AI tools for data analysts streamline analysis, funneling data into one solution for easier interpretation. Predictive AI forecasts future outcomes, aiding strategic decision-making. These AI-driven tools are indispensable for organizations navigating the data-centric aspects, ensuring competitive advantage and success.
Here is a look at the 10 best AI tools for data analysts:
Table of Contents
1. Julius AI
Developed by: Julius AI Inc.
Designed primarily for natural language processing tasks, Julius AI serves as a comprehensive tool for data analysts seeking to extract insights from textual data. Its user base ranges from individual analysts to large enterprises, offering robust capabilities in sentiment analysis, text classification, and entity recognition. Additionally, Julius AI integrates seamlessly with other AI tools for data analysts, ensuring enhanced functionality and efficiency in data analysis workflows, with high adaptability to diverse linguistic contexts.
Julius AI supports various file formats including CSV, Excel, JSON, and SQL databases.
Advantages:
1. Advanced Analytics: Julius AI offers sophisticated analytics capabilities, including predictive modeling and natural language processing.
2. Scalability: It can handle large datasets efficiently, making it suitable for enterpriselevel analysis.
3. UserFriendly Interface: The platform provides an intuitive interface for data exploration and visualization.
4. Automation: Julius AI automates repetitive tasks such as data cleaning and feature engineering, saving time for analysts.
5. Integration: It seamlessly integrates with other tools and platforms, enabling smooth workflow integration.
Read More: 15 Econometric Software Packages
2. Microsoft Power BI
Developed by: Microsoft Corporation
Power BI caters to a broad spectrum of users, from data analysts to business executives, empowering them to visualize and analyze data effectively. Its purpose revolves around data visualization, reporting, and dashboard creation, with extensive abilities in data modeling, interactive visualizations, and seamless integration with various data sources, including AI tools for data analysts. Power BI demonstrates adaptability through its user-friendly interface and scalability across organizations.
Power BI supports a wide range of data sources including Excel, SQL Server, Salesforce, and Google Analytics.
Advantages:
1. Interactive Visualizations: Power BI offers interactive and customizable visualizations for exploring data and discovering patterns.
2. Data Connectivity: It allows analysts to connect to multiple data sources and combine them into a single report or dashboard.
3. Collaboration: Power BI facilitates collaboration among team members through shared dashboards and realtime data updates.
4. Natural Language Query: Users can ask questions about their data using natural language, and Power BI will generate visualizations based on the query.
5. Scalability: The platform scales easily to handle large datasets and complex analyses, making it suitable for enterprise use.
3. Polymer
Developed by: Google
As a front-end JavaScript library, Polymer serves as a versatile tool for data analysts involved in building interactive web applications, particularly when combined with AI tools for data analysts. Its purpose lies in enhancing user interfaces and creating dynamic web components, catering to developers and designers seeking to streamline the development process. Polymer’s adaptability shines through its modular architecture and compatibility with modern web technologies.
Polymer can work with various data formats commonly used in web applications, including JSON, XML, and CSV.
Advantages:
1. ComponentBased Architecture: Polymer’s componentbased architecture allows for the creation of reusable data visualization components, enhancing development efficiency.
2. Flexibility: It provides flexibility in designing custom data visualization interfaces tailored to specific analytical needs.
3. Interactivity: Polymer enables the creation of interactive data visualizations that allow users to explore and manipulate data dynamically.
4. Integration: It integrates seamlessly with other web technologies and frameworks, facilitating the incorporation of data visualization into web applications.
5. Community Support: Polymer benefits from a large and active community of developers, providing access to a wealth of resources and libraries.
4. Akkio
Developed by: Akkio Inc.
Targeting data analysts, data scientists, and professionals in need of AI tools for data analysts, Akkio facilitates the automation of machine learning workflows, enabling users to build and deploy predictive models with ease. Its purpose centers on accelerating the model development lifecycle, offering capabilities in feature engineering, model training, and deployment, with adaptability evident in its user-friendly interface and integration with popular programming languages and platforms.
Akkio supports various data formats, including CSV, Excel, and JSON.
Advantages:
1. NoCode Model Building: Akkio allows data analysts to create predictive models using a visual interface, eliminating the need for coding.
2. Automated Feature Engineering: The platform automates the process of feature engineering, saving time and effort for analysts.
3. Scalability: Akkio can handle large datasets and complex modeling tasks, making it suitable for enterpriselevel analysis.
4. Deployment Flexibility: It offers flexibility in deploying models, allowing them to be deployed onpremises or in the cloud.
5. Integration: Akkio integrates with popular data analysis tools and platforms, facilitating seamless workflow integration.
5. MonkeyLearn
Developed by: MonkeyLearn Inc.
Geared towards text analysis tasks, MonkeyLearn appeals to users ranging from individual analysts to large enterprises seeking to derive insights from unstructured textual data, utilizing AI tools for data analysts. Its purpose focuses on text classification, sentiment analysis, and entity recognition, boasting advanced capabilities in natural language processing. MonkeyLearn showcases adaptability through its customizable workflows and seamless integration with various data sources and applications.
MonkeyLearn primarily works with text data in various formats such as plain text, documents, emails, and social media posts.
Advantages:
1. Text Classification: MonkeyLearn allows analysts to classify text data into predefined categories or tags automatically.
2. Sentiment Analysis: It provides sentiment analysis capabilities to determine the sentiment (positive, negative, or neutral) expressed in text data.
3. Entity Recognition: MonkeyLearn can identify entities such as names, organizations, and locations mentioned in text data.
4. Customizable Models: Users can train custom machine learning models tailored to their specific domain or use case.
5. Integration: MonkeyLearn integrates seamlessly with various applications and platforms through APIs, enabling easy integration into data analysis workflows.
6. Tableau
Developed by: Tableau Software
Widely utilized by data analysts across industries, Tableau excels in data visualization and exploration, empowering users to create interactive dashboards and reports. Its purpose revolves around simplifying data analysis and storytelling, with robust abilities in data visualization, ad-hoc analysis, and dashboard design. Tableau demonstrates adaptability through its intuitive interface and compatibility with diverse data sources. Additionally, AI tools for data analysts can further enhance Tableau’s capabilities, enabling advanced data processing and predictive analytics.
Tableau supports a wide range of data sources including Excel, SQL databases, Salesforce, and Google Analytics.
Advantages:
1. DragandDrop Interface: Tableau offers a userfriendly interface for creating visualizations, allowing analysts to build dashboards without coding.
2. Interactive Dashboards: It enables the creation of highly interactive dashboards and reports that allow users to explore data dynamically.
3. RealTime Data Analysis: Tableau can connect to live data sources, enabling realtime analysis and visualization of data.
4. Scalability: The platform scales easily to handle large datasets and complex visualizations, making it suitable for enterprise use.
5. Community and Support: Tableau benefits from a large and active community of users, providing access to resources, forums, and training materials.
7. IBM Watson Studio
Developed by: IBM
Positioned as a comprehensive platform for data analysis and AI development, IBM Watson Studio caters to data scientists, analysts, and developers. Its purpose encompasses the entire data science lifecycle, offering tools for data exploration, model development, and deployment, with capabilities in machine learning, deep learning, and AI-powered analytics, including AI tools for data analysts. Watson Studio showcases adaptability through its support for various programming languages, frameworks, and cloud environments.
Watson Studio supports various data formats including CSV, JSON, and Parquet.
Advantages:
1. Unified Environment: Watson Studio provides a unified environment for data analysis, allowing analysts to explore, prepare, and model data in one platform.
2. Machine Learning: It offers a range of machine learning algorithms and tools for building predictive models and deploying them into production.
3. Collaboration: Watson Studio facilitates collaboration among team members through shared projects and version control capabilities.
4. AutoAI: The platform includes AutoAI functionality, which automates the process of building and optimizing machine learning models.
5. Scalability: IBM Watson Studio can scale to handle large datasets and complex analytical tasks, making it suitable for enterprise use.
8. Alteryx
Developed by: Alteryx, Inc.
Alteryx targets data analysts and business users, providing a unified platform for data blending, advanced analytics, and process automation. Its purpose centers on streamlining data preparation and analysis tasks, with capabilities in data blending, predictive analytics, and workflow automation, including AI tools for data analysts. Alteryx demonstrates adaptability through its drag-and-drop interface and extensibility via integrations with external tools and databases.
Alteryx supports various data formats including Excel, CSV, SQL databases, and spatial data.
Advantages:
1. Workflow Automation: Alteryx allows analysts to automate repetitive data preparation and analysis tasks, saving time and effort.
2. Data Blending: It enables analysts to combine data from multiple sources and perform complex data blending operations.
3. Spatial Analytics: Alteryx includes spatial analytics capabilities for analyzing and visualizing geographic data.
4. Predictive Analytics: The platform provides tools for building and deploying predictive models without coding.
5. Scalability: Alteryx can scale to handle large datasets and complex analytical workflows, making it suitable for enterprise use.
9. DataRobot
Developed by: DataRobot, Inc.
Aimed at data scientists, business analysts, and AI tools for data analysts, DataRobot offers an automated machine learning platform for building and deploying predictive models at scale. Its purpose revolves around democratizing machine learning, providing tools for automated model selection, feature engineering, and deployment, with adaptability evident in its support for diverse data types and business use cases.
DataRobot supports various data formats including CSV, Excel, and SQL databases.
Advantages:
1. Automated Model Building: DataRobot automates the process of building machine learning models, from data preprocessing to model selection and tuning.
2. Model Interpretability: It provides tools for understanding and interpreting machine learning models, helping analysts gain insights into model behavior.
3. Scalability: DataRobot can handle large datasets and complex modeling tasks, making it suitable for enterpriselevel analysis.
4. Deployment Flexibility: The platform offers flexibility in deploying models, allowing them to be deployed onpremises or in the cloud.
5. Integration: DataRobot integrates with popular data analysis tools and platforms, facilitating seamless workflow integration.
10. RapidMiner
Developed by: RapidMiner, Inc.
Geared towards data scientists, analysts, and users seeking efficient AI tools for data analysts, RapidMiner facilitates end-to-end data analysis and machine learning tasks through a visual workflow environment. Its purpose lies in simplifying complex data analysis tasks, offering capabilities in data preprocessing, modeling, and evaluation, with adaptability demonstrated through its extensible architecture and support for various data formats and integrations.
RapidMiner supports various data formats including Excel, CSV, and databases.
Advantages:
1. Visual Workflow Design: RapidMiner provides a visual interface for designing analytical workflows, allowing analysts to create and modify workflows easily.
2. Predictive Modeling: It offers a range of machine learning algorithms for building predictive models, as well as tools for model evaluation and optimization.
3. Automated Machine Learning: RapidMiner includes automated machine learning functionality for automating the process of building and tuning machine learning models.
4. Scalability: The platform can scale to handle large datasets and complex analytical tasks, making it suitable for enterprise use.
5. Integration: RapidMiner integrates with various data sources and systems, enabling seamless data access and workflow integration.
Bottom Line
Hope you enjoyed our AI data analysis tools article. Please provide feedback.